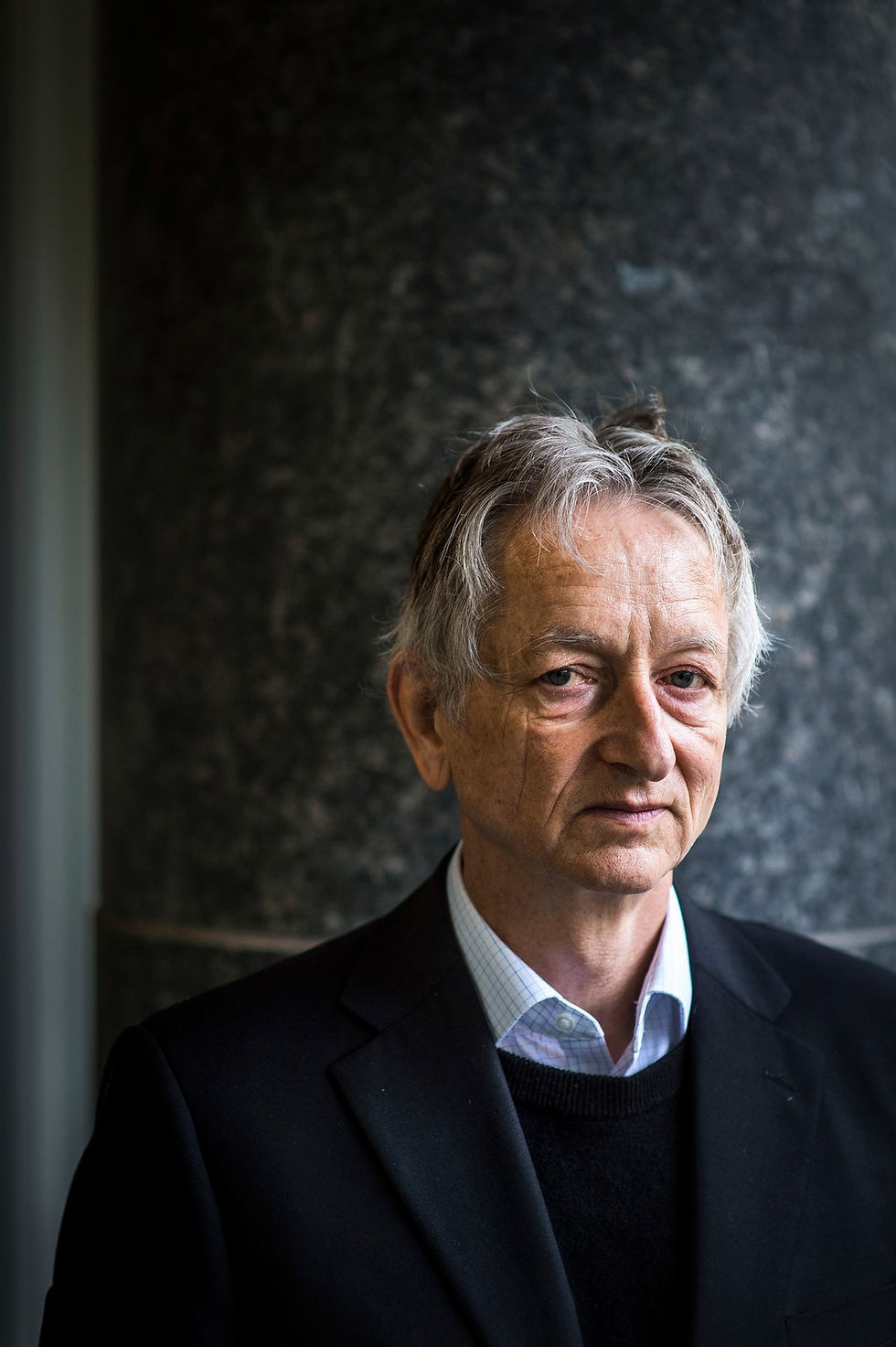
IN THE EARLY 1970s, a British grad student named Geoff Hinton began to make simple mathematical models of how neurons in the human brain visually understand the world. Artificial neural networks, as they are called, remained an impractical technology for decades. But in 2012, Hinton and two of his grad students at the University of Toronto used them to deliver a big jump in the accuracy with which computers could recognize objects in photos. Within six months, Google had acquired a startup founded by the three researchers. Previously obscure, artificial neural networks were the talk of Silicon Valley. All large tech companies now place the technology that Hinton and a small community of others painstakingly coaxed into usefulness at the heart of their plans for the future—and our lives.
WIRED caught up with Hinton last week at the first G7 conference on artificial intelligence, where delegates from the world’s leading industrialized economies discussed how to encourage the benefits of AI, while minimizing downsides such as job losses and algorithms that learn to discriminate. An edited transcript of the interview follows
WIRED: Canada’s prime minister Justin Trudeau told the G7 conference that more work is needed on the ethical challenges raised by artificial intelligence. What do you think?
Geoff Hinton: I’ve always been worried about potential misuses in lethal autonomous weapons. I think there should be something like a Geneva Convention banning them, like there is for chemical weapons. Even if not everyone signs on to it, the fact it’s there will act as a sort of moral flag post. You’ll notice who doesn’t sign it.
WIRED: More than 4,500 of your Google colleagues signed a letter protesting a Pentagon contract that involved applying machine learning to drone imagery. Google says it was not for offensive uses. Did you sign the letter?
GH: As a Google executive, I didn't think it was my place to complain in public about it, so I complained in private about it. Rather than signing the letter I talked to [Google cofounder] Sergey Brin. He said he was a bit upset about it, too. And so they're not pursuing it.
WIRED: Google’s leaders decided to complete but not renew the contract. And they released some guidelines on use of AI that include a pledge not to use the technology for weapons.
GH: I think Google's made the right decision. There are going to be all sorts of things that need cloud computation, and it's very hard to know where to draw a line, and in a sense it's going to be arbitrary. I'm happy where Google drew the line. The principles made a lot of sense to me.
WIRED: Artificial intelligence can raise ethical questions in everyday situations, too. For example, when software is used to make decisions in social services, or health care. What should we look out for?
GH: I’m an expert on trying to get the technology to work, not an expert on social policy. One place where I do have technical expertise that’s relevant is [whether] regulators should insist that you can explain how your AI system works. I think that would be a complete disaster.
People can’t explain how they work, for most of the things they do. When you hire somebody, the decision is based on all sorts of things you can quantify, and then all sorts of gut feelings. People have no idea how they do that. If you ask them to explain their decision, you are forcing them to make up a story.
Neural nets have a similar problem. When you train a neural net, it will learn a billion numbers that represent the knowledge it has extracted from the training data. If you put in an image, out comes the right decision, say, whether this was a pedestrian or not. But if you ask “Why did it think that?” well if there were any simple rules for deciding whether an image contains a pedestrian or not, it would have been a solved problem ages ago.
WIRED: So how can we know when to trust one of these systems?
GH: You should regulate them based on how they perform. You run the experiments to see if the thing’s biased, or if it is likely to kill fewer people than a person. With self-driving cars, I think people kind of accept that now. That even if you don’t quite know how a self-driving car does it all, if it has a lot fewer accidents than a person-driven car then it’s a good thing. I think we’re going to have to do it like you would for people: You just see how they perform, and if they repeatedly run into difficulties then you say they’re not so good.
WIRED: You’ve said that thinking about how the brain works inspires your research on artificial neural networks. Our brains feed information from our senses through networks of neurons connected by synapses. Artificial neural networks feed data through networks of mathematical neurons, linked by connections termed weights. In a paper presented last week, you and several coauthors argue we should do more to uncover the learning algorithms at work in the brain. Why?
GH: The brain is solving a very different problem from most of our neural nets. You’ve got roughly 100 trillion synapses. Artificial neural networks are typically at least 10,000 times smaller in terms of the number of weights they have. The brain is using lots and lots of synapses to learn as much as it can from just a few episodes. Deep learning is good at learning using many fewer connections between neurons, when it has many episodes or examples to learn from. I think the brain isn’t concerned with squeezing a lot of knowledge into a few connections, it’s concerned with extracting knowledge quickly using lots of connections.
WIRED: How might we build machine learning systems that function more that way?
GH: I think we need to move toward a different kind of computer. Fortunately I have one here.
Hinton reaches into his wallet and pulls out a large, shiny silicon chip. It’s a prototype from Graphcore, a UK startup working on a new kind of processor to power machine/deep learning algorithms.
Almost all of the computer systems we run neural nets on, even Google’s special hardware, use RAM [to store the program in use]. It costs an incredible amount of energy to fetch the weights of your neural network out of RAM so the processor can use it. So everyone makes sure that once their software has fetched the weights, it uses them a whole bunch of times. There’s a huge cost to that, which is that you cannot change what you do for each training example.
On the Graphcore chip, the weights are stored in cache right on the processor, not in RAM, so they never have to be moved. Some things will therefore become easier to explore. Then maybe we’ll get systems that have, say, a trillion weights but only touch a billion of them on each example. That's more like the scale of the brain.
WIRED: The recent boom of interest and investment in AI and machine learning means there’s more funding for research than ever. Does the rapid growth of the field also bring new challenges?
GH: One big challenge the community faces is that if you want to get a paper published in machine learning now it's got to have a table in it, with all these different data sets across the top, and all these different methods along the side, and your method has to look like the best one. If it doesn’t look like that, it’s hard to get published. I don't think that's encouraging people to think about radically new ideas.
Now if you send in a paper that has a radically new idea, there's no chance in hell it will get accepted, because it's going to get some junior reviewer who doesn't understand it. Or it’s going to get a senior reviewer who's trying to review too many papers and doesn't understand it first time round and assumes it must be nonsense. Anything that makes the brain hurt is not going to get accepted. And I think that's really bad.
What we should be going for, particularly in the basic science conferences, is radically new ideas. Because we know a radically new idea in the long run is going to be much more influential than a tiny improvement. That's I think the main downside of the fact that we've got this inversion now, where you've got a few senior guys and a gazillion young guys.
WIRED: Could that derail progress in the field?
GH: Just wait a few years and the imbalance will correct itself. It’s temporary. The companies are busy educating people, the universities are educating people, the universities will eventually employ more professors in this area, and it's going to right itself.
WIRED: Some scholars have warned that the current hype could tip into an “AI winter,” like in the 1980s, when interest and funding dried up because progress didn’t meet expectations.
GH: No, there's not going to be an AI winter, because it drives your cellphone. In the old AI winters, AI wasn't actually part of your everyday life. Now it is.
Source: Wired
コメント